Welcome to BioPortal
The world’s most comprehensive repository of biomedical ontologies
What BioPortal Does
Ontology Browser
Browse, find, and filter ontologies in our ontology library.
Term Search
Search for terms across multiple ontologies.
Annotator
Annotate your textual biomedical data with ontology terms.
Recommender
Get ontology recommendations for annotation based on descriptions of your datasets.
Mappings
Explore mappings between ontology terms.
Ontology Submission
Add your own ontology on any topic. (Requires free account.)
Ontologies
Classes
Users
What Users Say
BioPortal Products
BioPortal
Access and share biomedical ontologies on the web.
REST API
Use RESTful web services to access BioPortal content.
Virtual Appliance
Run our software as OntoPortal on your own system or in an Amazon Machine Instance.
Web Widgets
Use BioPortal technology directly on your website or in your application.
BioPortal is used all over the world

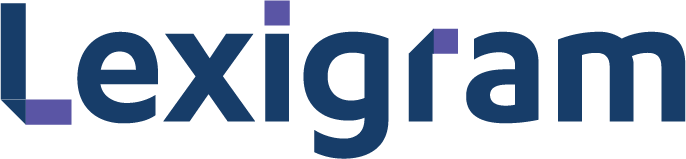

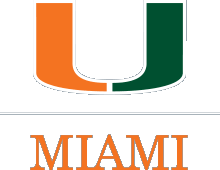

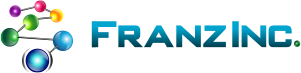
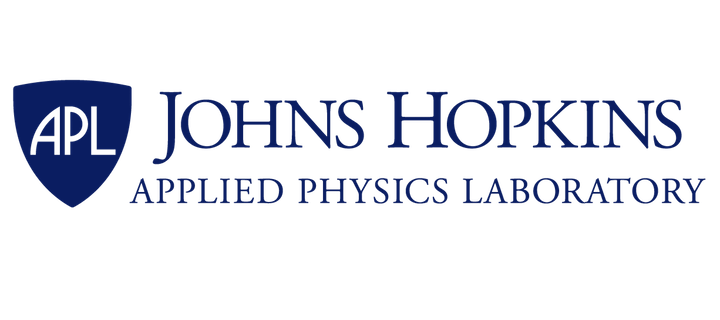
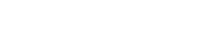
The National Center for Biomedical Ontology was founded as one of the National Centers for Biomedical Computing, supported by the NHGRI, the NHLBI, and the NIH Common Fund under grant U54-HG004028.
Copyright © 2005‑2023, The Board of Trustees of Leland Stanford Junior University. All rights reserved.